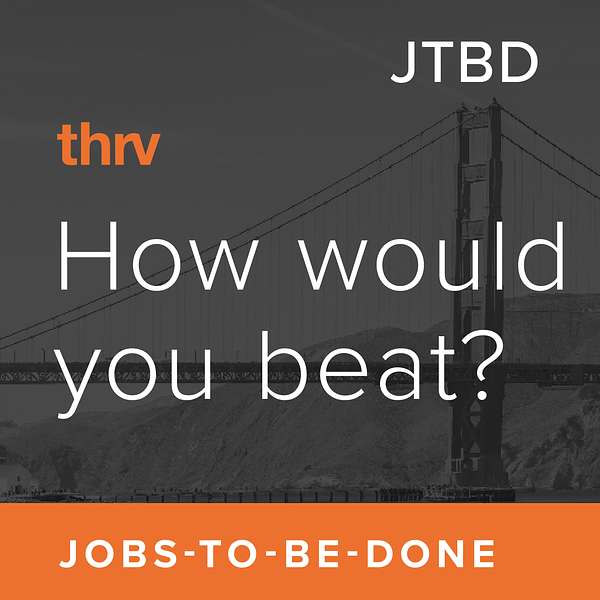
How Would You Beat?
A podcast that shows you how to use jobs-to-be-done (JTBD) methods to accelerate your growth and create equity value faster. This podcast is created by thrv.com for private equity CEOs, corporate executives, and product, marketing, and sales teams. Each episode explores how to beat your competitors to create equity value faster and with less risk.
How Would You Beat?
How Would You Beat Artificial Intelligence and Machine Learning Using Jobs-to-be-Done?
Artificial intelligence and machine learning are two technologies that have been improving quickly and definitely in the news. Google, Apple, Microsoft, Amazon, and every big company in China has been investing heavily in AI and machine learning. New companies like Open AI have received over a billion dollars collectively. Currently a lot of money is being invested in venture and in big companies in artificial intelligence and machine learning. So it better be worth it, there better be a return on that investment. So if you are on a product marketing or sales team, how should you use artificial intelligence and machine learning to accelerate your growth? And a good question is should you use it at all? How can Jobs-to-be-Done fit into the ML AI market? Let's find out...
✅ Download our Executive White Paper: "How to Use JTBD To Grow Faster" 👉 https://www.thrv.com/jobs-to-be-done-white-paper
Key moments from today's topic on how you would beat Artificial Intelligence & Machine Learning:
00:00 Intro to Artificial Intelligence and Machine Learning
05:23 Where would AI and ML land on your product roadmap?
08:29 Artificial Intelligence and Machine Learning being the solution to your customer's problem
14:29 Is AI the right tool for speed and accuracy yet?
19:33 ML and AI helping the emotion need of the customer
26:23 What platform do you choose to build Artificial Intelligence on?
✅ Download our Executive White Paper: "How to Use JTBD To Grow Faster" 👉 https://www.thrv.com/jobs-to-be-done-white-paper
Learn more about JTBD: https://www.thrv.com/jobs-to-be-done
Youtube: youtube.com/channel/UCEKeVWAeyQeMPzq9HfbUa5w
Linkedin: https://www.linkedin.com/company/thrv-com/
Twitter: https://twitter.com/thrvapp
Follow Jay Haynes on Linkedin: https://www.linkedin.com/in/jayhaynes/
Follow Jared Ranere on Linkedin: https://www.linkedin.com/in/jaredranere/
Welcome back to How would you beat where we discuss how you can use jobs to be done innovation methods to beat your competition. Remember to subscribe and like to this podcast. In this episode, we will look at how you could beat AI and machine learning artificial intelligence and machine learning, which are two technologies that have been improving quickly. And definitely in the news, Google, Apple, Microsoft, Amazon, every big company in China has been investing heavily in AI and machine learning. And even new companies like open AI, open aI have received over a billion dollars collectively, I think open AI itself has had about a billion dollars in equity funding, so a lot of money being invested in venture and in big companies in artificial intelligence and machine learning. So it better be worth it, there better be a return on that investment. So if you are on a product marketing or sales team, how should you use artificial intelligence and machine learning to accelerate your growth? And a good question is should you use it at all? So Jared, let's start with defining what artificial intelligence and machine learning actually are. What are they?
Jared Ranere:Yeah, great question broad one. But you could simply put it as artificial intelligence is the ability for a computer to solve a problem that we previously thought humans could. It you know, the historical classic test was designed by Alan Turing, and it's called the Turing test. And in an extreme case, the idea would be that if you were talking to a computer and couldn't distinguish whether it was a human or a computer, from the way it was using language and responding to your questions, then it passes as artificial intelligence. The definitions of that I think, have gotten more specific in recent years. So we now have notions of narrow AI, general AI and super AI. so narrow, artificial intelligence would be solving a very specific problem. We see examples of this when we use applications every single day. So for when you search for a mountain, in your Google Photos, and it gives you pictures of mountains, that's artificial intelligence, when you search for the name of somebody, you know, in Google Photos, and it gives you pictures of that person that that's image recognition, which is artificial intelligence, you could call it computer vision. It's a, it's a type of machine learning. And that's considered a narrow AI, general AI would be being able to solve problems that humans can do not just one specific type of problem. But anything you could throw any question at this, and Watson was considered to be a big investment in general artificial intelligence. The IBM is Watson, that one Jeopardy. And super artificial intelligence would be a computer that can answer questions that humans cannot questions that humans haven't even thought of, and that can teach itself faster than a human can understand. So those are the current definitions of artificial intelligence that I've seen widely.
Jay Haynes:Yeah, and so the question is, should you as a member of a company, as an employee of a company, working on a product development roadmap, for example? Should you use artificial intelligence or machine learning at all? And what is it useful for as you're building your product roadmap, you know, you've got to ultimately ship something to customers. And we see this a lot today that companies are touting their AI or machine learning. And there's clearly a lot of gray area between what is machine learning? And what is a true intelligence? You know, it has an interesting connotation that it's it is intelligent, and where is it just an algorithm that solves a problem and and that takes advantage of a lot of data that can help it the algorithm learn. And Rand has become something that's that obviously passes the Turing test. Now, that's not useful for product teams. Exactly. You're gonna have you're building a product roadmap, it's great for
Jared Ranere:doesn't matter if it's AI or narrow AR generally that like, who cares what you call it? And I think that's the crux of the whole argument, right? Do you put AI on your roadmap? Or do you put solving some important customer problem on your roadmap, and then survey the landscape to figure that out? And I have certainly been in situations where people have told me as a product manager, oh, where is this technology on the roadmap? I'm aware that there's a new technology in the world, or used to work in media and video was really growing at a rapid pace. Facebook had launched a bunch of video features, it was improving the number of visits and all their usage metrics. So the call question for everybody who worked in internet media was What are you doing with video that happened with mobile phones? When the iPhone when the app store got really big it was what are you doing with mobile? And this question comes up to product managers all the time. So how would you handle this question? If let's say, you were working at Google? Isn't it do something with it? It's a great
Jay Haynes:question. Because if you're using product roadmapping tools, you and you're trying to answer this question, it's very, very hard to do. There's a lot of product roadmapping software, there's a lot of product roadmap and tools, and they're very, very, very good at some things. Obviously, they help teams, you know, stay coordinated. But if you were using those product roadmap tools to try and answer this question is where does AI or machine learning show up in our roadmap, it'd be very hard to do. And the reason it's hard to do is because any product roadmap at the end of the day, the reason to put something on your product roadmap is it's going to create value for your customer. You know, you don't want to ship products that don't create value for your customer. Otherwise, you know, you end up with Microsoft Zune, or you end up being Blackberry or Kodak or you know, all the examples we use. So, the key is then assessing the why, which is why with AI or machine learning create value for our customer. And this is, of course, why speed and accuracy and cost are so important. Because if you're saying, let's look at our product development roadmap, and first ask our question, ask ourselves the question, why are we building this stuff on our product roadmap? What is the reason? And if you have a good reason, and it makes sense, great, you know, fantastic, you know, all the all the product roadmapping software use would be great, because you could put all that in there and just have a justified product roadmap, and you're on a path to success. But if you really are trying to figure out quantitatively, what the value you're going to deliver in a roadmap is if you ask that why question, that's where you get to ultimately the customer problem you're trying to solve, which you know, these days, called the customer job to be done independent to the solution. So then you can map any algorithm, whether it's artificial intelligence, or whether it's, you know, machine learning, or whatever you want to call it, anything that delivers more speed and accuracy to your customer in solving the problem and getting the job done. That's going to create value. And what I find fascinating, you know, Jared, we see this all the time, obviously, is that you don't need a lot of intelligence in so many markets to create value, because the way customers are getting them, the job done today is still incredibly manual. In other words, if you're if your competition is someone manually creating PowerPoint presentations, or using spreadsheets, you definitely don't need artificial intelligence to great value, which is really good news. Like you don't have to, you know, cure cancer in order to to, to accelerate your growth.
Jared Ranere:Yeah. And I think one way to approach this, which we've talked about another podcast is to think about, imagine the solution to the problem was a push button, you just click the button, and the problem was automatically solved, the whole job got automatically done. How would we achieve that? And today, you anytime you have that question, you want to survey the landscape of the landscape of available technologies. Right? So while we could put it on the internet, we could put it on a mobile phone, would that help? We could, you know, have a database with a bunch of information in there that is delivered to the user? We can What if we add artificial intelligence on it? Will it solve a problem and enable our customers to push a button and get the job done? So what's great about a lot of the AI out there today is that it's becoming more and more possible, right? Instead of it being a pipe dream, that you can push a button and get the job done. The technology is advancing at such a fast rate that that's becoming more and more true. One example of this is writing copy, you know, just creating sentences. For example, for marketing purposes, there are companies like Jasper AI, and a host of others that are using large language models, which is a type of artificial intelligence to automatically create marketing copy. And that seems like a pipe dream, right? If you could, if you could just say like, Hey, create marketing copy from our product and instantly, you know, five years ago, you would say there's no way to do that. We can give prompts, we can suggest ways for people to write. But now it can actually be written for you, which is kind of amazing. So it's about creating a very specific problem. What is the job What is the struggle the customer is having within that job, and then surveying the technology landscape and figuring out how to apply these things?
Jay Haynes:Yeah, I think that's such a good point. And the, the reason it can be so valuable is because every single customer problem, as we call it, the job to be done is basically a system where you've got to get information, and you've got to make decisions, and you got to see how the job is going, assess it and revise it, and then ultimately conclude the job. So you can apply this to getting a baby to sleep through the night, right. Or you could apply it to getting to a destination on time, or you could apply it to, you know, knee surgery, or living with diabetes. So, and in each of those cases, you need information. And that information, you know, resides all over the place. So some of the machine learning or the AI can relate to just getting the right information and figuring out, if it's the information that's going to lead you to successfully getting the job done. And I'll give you example of getting a baby to sleep tonight, is there's a lot of information out there that is incorrect, that will absolutely lead here baby not sleeping through the night. Just the same way, I'm sure there's information about knee surgery, you know, or accounting that is inaccurate. Alright, so the I could go out there and say, Okay, well, let's get the right information, make sure it's correct. And then you have to make all sorts of decisions, you know, your baby didn't sleep well, this night, you need to change the schedule, the feeding schedule, or the knee surgery is going in the wrong way. Or you need to optimize cash flow, your business, you know, whatever, whatever the goal you're trying to achieve is could be going off track. And you need to use that information to make that right decision. So people are overwhelmed by information and decisions they have to make, you know, if you just go through what like a typical person living in the world in 2022, it's just an enormous number of decisions to add in your parents and you know, then you're out in your career and your work. And you know that the other things you want to do with your life, like we don't plan for retirement or have some hobbies, it's just we're incredibly busy and making these decisions. So the value comes from Ken, the technology, it doesn't even matter if it's AI or machine learning, can the technology first and foremost, deliver better information and better decisions today? And then what I would say is, can you figure out how to continually improve that speed and accuracy of whatever it is an algorithm or machine learning, that's the idea behind machine learning is that you know, it's essentially going to process things and figure it out, which which photograph is a mountain, right? I don't think it's specifically I would classify that as intelligence. It's a, you know, advanced categorization that humans do with ease, right, because every human looks at a picture of mountain and we have it automatically figure it out. So yes, that's a that's a, you know, super important technology that's progressed to an impressive degree. But you have to then apply this kind of thinking to your roadmap, and what your what your product development roadmap should be doing, because you know, the job is the same. And so your customers problem is not going to not going to change dramatically, you know, it's the same job. That's the whole theory is that it's stable over time. But that technology's ability to get the job done faster and more accurately, is going to change dramatically. And that's where you really want to stay on top of what's happening in AI and machine learning. Because if it applies directly to your customers job, your competitors are going to figure that out. And no matter what, like product roadmapping software using, or whatever is in your roadmap, if you're not aware that open AI or you know, Google's algorithm and a AI or IBM is, you know, there's a ton of companies that are building this kind of API system and be able to make calls to their AI and figure something out. You just have to know like, what is the problem you're solving for your customer with that, but you have to stay aware of it, because your competitors will use it at some point. Right? That is where the market opportunity intersects with the technology, because people customers will switch to something is faster and more accurate. That is true throughout, you know, human history. Yeah. And
Jared Ranere:I think that AI presents an interesting question about speed and accuracy, where in a lot of cases, it's a lot faster, but not very accurate. In some cases, it is very accurate and faster, right. But you have to figure out like what is the balance for my job that makes the most sense for my customer. So for example, in the Google Photos example, if you're trying to find a photo of a mountain and one of the photos in there is of a model volcano from the science fair It's not really a big deal, it's a very low stakes job that you're trying to get done for your customer, I can just flip by that photo and like life doesn't really change. If I'm trying to do things like mint tried to do where there were categorizing expenses, for my personal finances, and you know, you can think about this QuickBooks might be trying to do this, for bookkeeping purposes, to file taxes, and run your business and figure out what decisions you should make to optimize your finances to run your business or your personal finances to live better. The stakes are much higher, you know, if if something gets classified as a business expense, which is not an I take a tax write off for that. And if it's large, that there's there's high stakes there that I don't want that to be incorrect. And you saw that with mint. You know, whether or not mince machine meant to if anybody doesn't remember, mint was a piece of software, where you could connect your bank accounts and your credit cards, and would automatically categorize your data and give you insights into how you were spending your money, and then make recommendations about you know, new offers for credit cards, loan products and investment vehicles that would help you achieve your financial goals. Now, when I tried to use that the categorizations were so bad, that I had to spend so much time correcting it, that it just became completely useless. And that's an area where you might say like, you know, like, it's, maybe AI is not right for this, it's not good enough yet, that, you know, making it easier for the user to classify themselves as it goes, is is a better experience than having all these things be totally wrong and having to go in there and correct them or forget to correct them, because you thought it was automatically done. And now you've got terrible decisions happening downstream because you're working with bad data. So I think this is a really interesting area where it's important, think about what are the stakes of the problem that you're trying to solve? And how accurate does the solution have to be?
Jay Haynes:Yeah, and I think I think that's right, because if your product roadmap is in a market that's, you know, geared towards entertainment. You know, entertainment isn't as high stakes. You know, for the on the consumer side of it, obviously, if you're investing in a movie, you know, that that's a high stakes, lot of capital. But, but I think you're right, and so if your roadmap is actually really meaningful to your customers, in other words, the problem you're trying to solve is something like personal finances, personal health, even parenting, obviously, there's huge categories where the stakes are very high. And you want to be able to prove that the value of the AI or machine learning is over and above what this current state of the art is. And that I think, is really the key. So even if Mensa good example, like if they can enhance their algorithms that categorize stuff even a little bit, they can then measure you going through and doing it on your own, which could take a long time versus their algorithm doing it. And if it if it's can get to the you know, pretty good accuracy, but very, very quick. And then you only have to make a few changes. And then that can be an interesting UI to that they could put in front of a user and say, here's our automated version of this. Could you please check on it and have a nice interface to yes, no, this is correct or no. And then that input actually becomes part of the learning algorithm. And this is the way you know, not being a machine learning expert. But I do know enough about my theory that those inputs help give the the algorithm, its ability to get better. That's the learning idea, or the intelligence idea, you know, depending on where you draw the line. Yeah. But I think that's really valuable, that companies can start there and say, okay, as long as we're, we're in the game, of continually improving speed and accuracy, that's how your roadmap and you know, all the stuff that you're doing in product development and putting in a roadmap, all that is really, really going to pay off over time. Right? Because that that, again, it will be speed and accuracy that wins the day. That's why all innovations succeed.
Jared Ranere:And I think that there's a there's an interesting emotional need there too, which something we don't talk about as much. But you what you need the confidence as a user that your problem is being solved accurately. Right. So let's say you have hundreds of transactions that are being classified by mint. How do you know if they're right? How do you how do you know if you should be confident or not without reviewing them and what's The interface that helps you build that confidence. Because if you don't feel that you won't trust it, you won't continue to use it. And this is a really interesting area where that implicates how you design your user experience. Because it could be that your algorithms 100% accurate. But how do you help the user believe that? If they don't believe it, then it doesn't matter, they'll stop using it, or they'll do the work and it'll be slower for them. So it's the end this, you see this a lot in chatbots, to where it's, you know, the, what is the saying the right thing for a chatbot. So a chatbot, being, you know, when you let's say you're on a website, and you're on your mobile providers website, right, you're on Verizon, and you've got a billing issue, and you chat with a little window, and you say, Hey, I've got a billing issue, and they have some automated response that's generated by a robot, not a real human. What is the right response? What are you trying to make people feel? How, how do they trust, the voice behind that chatbot there's a lot of emotional questions in there that, that get raised when it's, the user is not doing it for themselves, but it's being automated for them.
Jay Haynes:Yeah, and that's, I think that's a great point is even the best product roadmapping tools don't have this emotional component. Because they don't have the perspective of the customer and their struggle. And that's, that's where I think they're useful for a lot of things, but not this kind of customer empathy, where you're saying, Okay, we need to design this product, let's envision what our customers going through. And the simplest way that you can do this, that we, when we work with companies, we tell them this is that you can imagine a scale from your customers incredibly anxious about getting the job done successfully. A good example would be like parent trying to get a baby to sleep through the night. Anybody has been a new parent, all the way to extreme confidence. And you can imagine things that have built that emotional response and getting the job done that we use every day, you know, Apple and Google Maps, we use that example all the time, I used to live in Southern California, where you had a Thomas guide, and he told you absolutely nothing about the traffic driving around Southern California, which is gigantic, and you're always on the road, you know, you have to flip this map and go through four different pages of maps to figure out where you're going with no idea if you're going to be on time, you know, it was really, it's really anxiety, really, and the only solution was to like, leave an hour early. And now you hop in, you know, Apple, and Google Maps your ways, they pretty good job, you know, the plus or minus, you know, certain percentage of time, that's the accuracy, they really do tell you, you're gonna, you know, you're gonna be there at 315. Right. And that's a much more confidence inspiring experience, which is an emotional relationship, because they are successfully getting the job done. They really have speed and accuracy nailed like you, you're pretty confident if you use those applications that you're going to arrive within the right amount of accuracy.
Jared Ranere:There's a great parenting product, as that's an example of this, this anxiety situation and how you have to be very aware of it. I cannot remember the name of the product, but it essentially tries to measure your infant's breathing while in bed at night, and you have a monitor that tells you when the breathing is erratic, or in some way. And the only anecdotes I've heard about this from people who have had it is false positives, which are the most anxiety riddled moments in a parent's life when they have a false positive that their child's breathing is erratic, and they race into the room. And they it turns out the kids three in the morning. Yeah, three. That's right. That's right. They're woken up by this alarm, right? Raising the turns on the child's fine just sleeping, but they like rolled over or something or you know, who knows what, maybe like, coughed on a little phlegm or something like that. But there's no real severe issue. Meanwhile, you've woken up a kid because you've raced in there, and maybe you've slammed the door or something. And now you've got a screaming kid. And I mean, this is not just like, not only does it not get the job, but it makes the job a whole lot worse. Yeah. So really think about that
Jay Haynes:it really is important. And that's a good example of where machine learning could be really, really useful to them as they are working on therapy, again, their product relevant roadmap. So the idea of a platform that does monitor for I think it's called SIDS, right Sudden Infant Death Syndrome. And it scares every new parent right? Because oh my gosh, it's it's it is a nonzero percentage of kids who just you know, essentially die in their crib, right because they stopped breathing. And, and there's all sorts of other stuff around that too, not just the monitor like you're not supposed to have like bumpers on the cribs, I think are really bad,
Jared Ranere:you know? Yeah, no blanket or stuffed and
Jay Haynes:they're just swaddled and no pacifier. Yeah, there's a whole system of things to scare parents today. Because it is terrifying. Obviously, it's like the worst possible thing that could happen. So they're in the right market, I would say that, that job of preventing SIDS and you know, ensuring your baby's health broadly, the domain is really, really important. And they're on the right track. But I think where you've, you've gotten some good customer feedback, like false positives are not good. It's inaccurate and more terrifying than it needs to be and makes it it also doesn't make you question is going to detect the positive thing? Because it makes you question like, is the thing working, you know, at all? So that's a case where machine they're on the right path? Right, that that is a that's a true market? I bet that you mean, I don't know the company, but I think it's probably, I'd be surprised if it's not selling some of those products. Because that's a real parenting need and job. So, but But yeah, a good example of where they should be evaluated, they probably are evaluating all sorts of AI machine learning, like what can improve that very quickly, so that it eliminates false positives and gives you much more confidence.
Jared Ranere:Right. Right. Right.
Jay Haynes:Yeah, and that's the key, you know, the, the best way to do this is to start with understanding the problem. And then evaluating any technology, it doesn't matter if it's AI or machine learning, or, you know, whatever you want to call the new algorithms that people are going to use, you know, broadly, they fall in those categories. But it could just be a straightforward algorithm doesn't have to be all sophisticated, that it's going to create customer value. And I really do think that that can help product teams that have to build roadmaps that are responsible for these trade off decisions that are responsible for, for timing, because the you know, all the product, roadmap, software, and tools really do help you figure out timing and prioritization, because that's where you want it the Should we do it now? Should we do it next quarter? Should we wait a year? Should we monitor it, you know, these are incredibly important, ongoing decisions. And this stuff is changing incredibly rapidly, even open AI, their large language model, which Jared, I know, you and I have discussed going from version one to three. And I think for very soon, you know, obviously, behind the scenes, Google and Apple, Amazon, IBM, are doing very interesting stuff that's going to impact product development roadmaps all over the world, because this stuff is being delivered in API format. It's not, you know, it's actually really interesting that this is how it's emerged rather than just, and of course, companies have their own AI, you know, Apple is building its own stuff that is, you know, just just for Apple, like face ID and, you know, all sorts of other algorithms are building that are proprietary. Unfortunately, I don't think they've applied enough of that to Siri, I would love series. More intelligence, I would classify Siri is intelligent yet. Yeah. So but it is emerging as, as this class of API's where you can access the sophisticated intelligence as a as a company building a product development roadmap, without having your own product development roadmap for AI. And that's what I think is so interesting. Like, you don't have to if I mean, if you're Google, great, I'm sure you've got, you know, teams of people working on this. But if you're XYZ quo, you still are in the market for getting your customers job done faster, more accurately. And you can use the all of the r&d research into AI and machine learning, as long as you use some sort of a, you know, product roadmapping tool to assess where the AI is going to deliver value to your customers.
Jared Ranere:Yeah, it's almost like the question is not becoming, are you building any AI into your system? But which platform? Do you choose to build it on? Which API do you use? Which tools within that? I mean, once you start to get into this, there are a host of different ways to approach using machine learning, natural language processing in your application, and there's a lot of decisions to be made once you go down that path. And so it's a you have to make a bet long term, medium term and short term on how you're going to impact your roadmap and run experiments with you know, you're betting on a platform you're betting on API, how fast is going to advance how open is it going to be? Can I continue to use it? Is it going to be a competitive threat at any point? And that's, that's really interesting. You have to end up being quite old. Ben to make a core part of your application, you know, algorithm that somebody else owns and runs.
Jay Haynes:Yeah. And, and you can do both. You can be using whatever in the short term is going to create value while you build your own, if that's, you know, in your product teams ability and your engineering team's ability, which, which is great like, and that still helps you with this roadmapping issue, which is to do with short, medium, long term short term might be, you need to get into the market with it, because there's an API you can use, it doesn't really well, longterm, maybe let's build our own because we're, we're so specifically focused on our customer's job that we want to fine tune the algorithms to this job. And I think that's really, the key and probably a good place to end is that when you're evaluating stuff, you're looking for inputs and outputs, that's really the idea. It doesn't matter if you're trying to get a baby's sleep tonight, prevent SIDS, you know, perform knee surgery, you know, ensure aircraft airworthiness, you know, get to a destination on time, you need to know the set of variables that are the inputs, you need to know the decisions that need to be made and the outputs. So a good example of this, which we talked about all time is getting to a destination on time, one of the needs and planning the stops is to determine the optimal sequence of stops. And so that's a good example of where you have inputs you need, which are the stops, and you need to know what time you need to be at the different stops or your meetings, etc. You need to know the optimal sequence of those, which is actually called the traveling salesman problem. But that's the decision you didn't make am I going to be on time in my busy day as I have five different meetings throughout a you know, geography that I don't know that well, and there's a ballgame at three o'clock when my right when my meetings there, those those are the types of things that are the variables, the inputs and outputs. And you can tell if an algorithm is going to do it better if you have the needs specifically in mind with the job step and the job itself. And that's what's really, really powerful is you can test this out and say, Okay, today, you know, open API's. API is doing a great job in this today. So let's use there for a short term, because all we have to do is make some API calls, and we can get the answer we need, we can help the customer make the decision they need to make which is getting the job done. And then from there, okay, well, let's build our own optimization algorithm because we know we're going to be focused on that and what is that going to look like? And then you can have these parallel product development roadmaps that are one with the API to your own internally, ultimately, to create the most customer value at the end of the day. That's what it's about. Do you help your customer get the job done faster and more accurately, right. Yeah, great, great place to stop. Thanks for listening. Remember to subscribe and like this podcast. If you want to learn more about jobs done innovation methods, visit us@thrive.com that's T H R v.com.