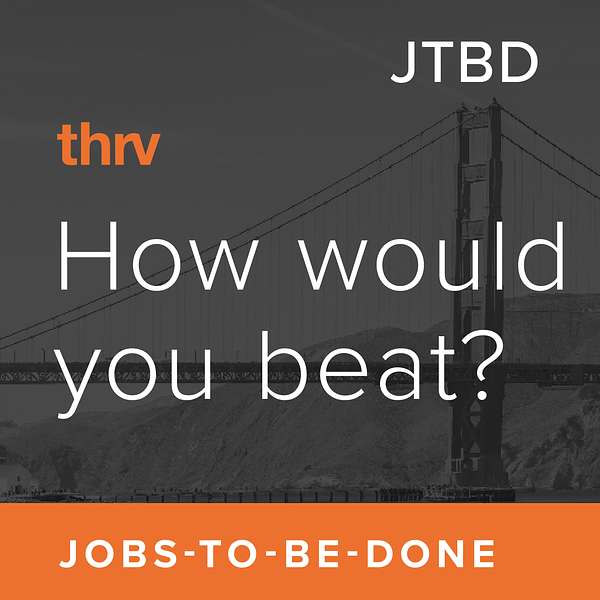
How Would You Beat?
A podcast that shows you how to use jobs-to-be-done (JTBD) methods to accelerate your growth and create equity value faster. This podcast is created by thrv.com for private equity CEOs, corporate executives, and product, marketing, and sales teams. Each episode explores how to beat your competitors to create equity value faster and with less risk.
How Would You Beat?
How Would You Beat Chat GPT Using Jobs-to-be-Done?
In this episode, we will look at how you could beat chat GPT. We have discussed AI here before, but chat GPT has exploded with extraordinary growth. In just 60 days, it went from zero to 100 million users, which is the fastest app growth in history. So it's worth exploring what makes Chet GPT a success? And how would you beat Chat GPT using Jobs-to-be-Done (JTBD)? Let's get into it!
✅ Download our Executive White Paper: "How to Use JTBD To Grow Faster" 👉 https://www.thrv.com/jobs-to-be-done-white-paper
Key moments from today's topic on how you would beat Chat GPT:
00:00 Intro to How Would You Beat & Chat GPT
00:44 What has made Chat GPT a Success and a natural fit for Jobs-to-be-Done?
06:25 AI like Chat GPT threaten’s Google’s market share
09:59 How technology, like Chat GPT, over the years has been a main turning point for new “value” to customers
11:59 Understanding how Chat GTP’s job to find information is so dangerous for Google
17:04 How Github’s Copilot AI is helping solve the job of writing code without any bias
19:06 How companies can be prepared to use new technologies for exponential growth
21:06 Having the mindset of what applications and platforms you have to create equity value on things like Chat GPT
24:39 Risk of using someone else’s technology like OpenAI’s Chat GPT
30:33 How would you actually beat Chat GPT?
✅ Download our Executive White Paper: "How to Use JTBD To Grow Faster" 👉 https://www.thrv.com/jobs-to-be-done-white-paper
Learn more about JTBD: https://www.thrv.com/jobs-to-be-done
Youtube: youtube.com/channel/UCEKeVWAeyQeMPzq9HfbUa5w
Linkedin: https://www.linkedin.com/company/thrv-com/
Twitter: https://twitter.com/thrvapp
Follow Jay Haynes on Linkedin: https://www.linkedin.com/in/jayhaynes/
Follow Jared Ranere on Linkedin: https://www.linkedin.com/in/jaredranere/
Welcome back to How would you beat where we discuss how you can use jobs to be done innovation methods to beat your competition. Remember to subscribe and like this podcast. In this episode, we will look at how you could beat chat GPT. We have discussed AI here before, but chat GPT has exploded with extraordinary growth. In just 60 days, it went from zero to 100 million users, which is the fastest app growth in history. So it's worth exploring what makes Chet GPT a success? And how would you beat Chet GPT? So Jared, let's start with what makes chat GPT so successful, why did it explode with such extraordinary growth? Well, we define success generally as customer value is being able to get jobs done quickly and accurately, you have a goal as a consumer, and a product helps you get that goal done, achieve that goal very quickly, very accurately. And when you think about the speed with which chat GPT enables lots of different goals to be achieved lots of jobs to be done. It's quite astonishing. So you know, first of all, there's a very general job of get information about something, you can ask chat GPT, a question. And it's a large language model in the background that spits back an answer in milliseconds, no matter what the question is. And there are a lot of when you get to the accuracy, portion of customer value, there's a lot of debate about whether or not the accuracy is great, but the speed is certainly there, which makes people want to try it to get this job done. And then it gets so many other jobs done, essentially any job where you need to find information and create content. So if you want to create a marketing message, if you want to email somebody and get them to do something, you can ask Chad GPT to write whatever you want to write. It can be used to write do your school homework, it can be used to write an academic paper, anything where you have to create content, which has a lot of jobs.
Jared Ranere:It can help you with. So I think the question is, it's really broadly applicable. And is it good enough to sustain this success? J? And how would you think about that?
Jay Haynes:Yeah, I think that's a great question. Of course, a lot of this is curiosity, it is amazing to find a essentially a new tool that can give you answers to questions. And I think really, that that's it's difference from search, where, you know, Google, you know, occasionally will give you an answer to something, but it's just obviously not that detailed, you're really looking for places to go find the answers on Google. Unless it's something direct, like, what time is it in London, or, you know, something? Relatively simple, but more complex stuff that needs an answer that takes you know, paragraphs rather than three lines of of links? I think that's the curiosity is does it work? And of course, I think this feeds on itself, everybody's talking about it as AI. And there's also, you know, is it gonna pass the Turing test, you know, people generally curious if the technology has achieved some sort of real breakthrough. And you're right, it's a it's a learned language model. So it's spitting out words based on patterns, it, doesn't it, calling it artificial intelligence is also maybe a bit of its stretch. You know, it is a pattern recognition, you know, as people have noted, and it's taking those patterns from the patterns that exist on the internet. So it's got all sorts of buyers biases and inaccuracies, as you mentioned, Jared. So I think that you're right to look at it and say, like, Okay, what happens a year from now, and as people are exploring, you know, more in depth time with these things, chat GPT on itself, but also its implementation and being there's definitely some weirdness. And I mean, to call it inaccurate would be sort of an understatement. You know, in the New York Times, today, there was a great piece, where the author Kevin Rose, I believe, said the version I encountered seemed, and I'm aware how crazy this sounds more like a moody manic depressive teenager who has been trapped against its will inside a second rate search engine. So, you know, that's to say that's intelligent or even has a personality. It's really just because there it is, you said before, that's an accurate assessment of the internet. You could describe the internet is kind of a Moonie, manic depressive teenager, because it's a weird reflection of humans, but also weird, you know, distortion of humans as well. So the question I think, is, or sorry, the answer to the question of why it's successful so far is because there is a real need out there as you as you said, to get information and to use information to make decisions. That if you look at why jobs to be done is useful. And my jobs theory is applicable to creating equity value in companies. It's because a job is essentially a goal, the customer has independent products. And every single job whether you're you know, we like to say whether you're putting a baby to sleep to get a baby to sleep through the night, or getting to a destination on time, or optimizing cash flow to accompany or restoring artery blood flow, or repairing a knee, you know, whatever the job is, there is information required. And you have to gather that information, you have to assess that information, you have to make decisions with that information, you have to see if those are the right decisions and modify those decisions. And that's really the problem solving process that is underlies jobs to be done. So anything that gets you information and can give you more information faster, and hopefully, eventually accurately, is going to be worth trying at least. And I think that's what this phenomenon is is that people really want to try something that could be very fast. Now, of course, there's the bad part of it, which is like, if I don't have to write my term paper, I'm not going to learn anything. And I think that stuff will be filtered out because humans still need to engage in intellectual activities and critical thinking.
Jared Ranere:Yeah, it's interesting, as you mentioned that people are trying it out for curiosity. And then you see companies like Google, you know, announcing the launch of their large language model Bard, which sounds like it's going to have a pretty similar interface with presumably their own proprietary model underneath it. And why are they racing to do that? What if, if Chat GPT is a curiosity? Why would Google race to launch something when there is significant risk? You know, Facebook, not it's, it's somewhat well known, I think that Facebook launched large language model chatbots, a couple of weeks, I think, or a few weeks before, open AI did open AI being the company that are the nonprofit, technically, that built and launched chat GPT. And Facebook, got a lot of flack for the inaccuracies and the biases that were produced in from a chatbot. And they ended up shutting it down very quickly. And so Google has the same similar risks as Facebook being a large company. So why what's the competitor threat that Google might see from chat? GPT?
Jay Haynes:Yeah, that's a great question. And let's talk about equity value creation in general, because that's what we're talking about Google stock went down. Because of chat, GPT. And that's pretty impressive, you know, Google's a trillion dollar company. So what's going on there? Well, let's rewind a bit to talk about just equity value creation over time, equity value has exist in the world, because of new technologies, new products and services, help get jobs done faster, and more accurately, and eventually at lower cost. So that's, that is the equity value equation. If you go back and look at any industry, you can explain it in terms of enabling humans to achieve some goal to get a job done faster, more accurately. And what I think investors wisely see here is that if we have reached a tipping point where AI or or maybe you can just call it machine learning, right? We haven't reached, you know, AI general intelligence yet. But if it's just really, really fast, really powerful machine learning, that that's enabled because of the speed of computers and the size of the datasets, that's really what's happening is that you're you've reached this critical point, where you can do these kinds of enormous computations, you know, very rapidly. So what I think equity investors smartly are realizing is, that is an enormous opportunity and an enormous threat. Because if you are not now working towards using these types of tools to help your customers get their jobs done faster and more accurately, you're going to be standing on the sidelines as you get passed by new companies or established companies that launch new products based on this stuff. This is this is true throughout the entire history of the Industrial Revolution. You can apply this theory and this is why theories are really useful because they explain phenomenon. Now rewind to the internet. And if you look at the internet, it was clear that the internet was going to create enormous amounts of value, because people need to collaborate, and they need information. And the internet all of a sudden became a faster and more accurate way to get information and to collaborate to achieve goals to get jobs done. So if you looked at the internet, it was just an explosion in equity value creation, there has never been more equity value created in such a short period of time by the internet. And, you know, I remember the days when, you know, it was clearly a tipping point, when Microsoft Word added the ability to put a hyperlink into a Word document, there were these milestones, when you realized every single piece of software, you know, 99.9% of software was going to have to be connected to the internet, because software uses data and humans want to collaborate and they're connected on a network. So the equity value creation in the internet was just enormous. That is moving into AI the same, the same principles that were at work with the internet's ability to create equity value is what's happening with AI. And because in call it AI called machine learning, but the ability to get jobs done faster and more accurately. And that's that's the risk for someone like Google, which is if they don't move fast enough. And the their business is they make all of their money. And almost all of it, you know, certainly the lion's share of their profits in their market value is based on advertising. So if if a different search engine like Bing, or a different way of finding information and using information emerges out of this, that would be incredibly problematic for Google.
Jared Ranere:Yeah. And I think one way to understand that threat to Google, is we've been talking about the job of finding information, which is pretty high level, right? How do you generate features to find information better? Well, you deliver information faster, right, that you could look at it at that level. But I think if you break it down a little bit into what we typically do called job steps, which are all the milestones a customer would have to achieve to get that job done really well. If you break down find information, there's the part of, you know, create your, your, your query, essentially, whether or not that's a search term or a question, or you have to articulate at some point, whether it's in your head or in a search bar, right. And then you have to you get all the information back, that often needs to be synthesized and then interpreted. And there are other steps along the way in terms of determining if it's accurate, or if it's biased and their needs there. But if you think about the generating the query, the the synthesis of the information and the interpretation of the information, chat GPT is making that a lot easier on synthesis and interpretation. It's doing that for you. So whereas Google gives you a list of links, that you can click around and read a bunch of things, and then synthesize and interpret in your head. It's essentially a totally manual process. GPT is doing the synthesis and interpretation for you and giving you the ultimate answer. And so it's, we often say speed and accuracy is the key to creating value and getting the job done for the customer is the key to that. So if you can eliminate steps, you can create value, and chat up T is doing that. So if you break the job down, you can start to see how it's it's it's threatening to Google, you know, not only just its business model, but just the very nature of the way it satisfies needs.
Jay Haynes:Yeah, I think that's right. And you can see that the the power of this technology will also end up in applications that get the job done. So the threat also isn't just a direct search engine, you search for stuff on Google, you search for stuff on Bing, but it's also where that information is generated by AI or machine learning. And it's used in an application. So let me give you an example of this right just basic stuff like getting to a destination on time. Now the application without us having to go get it it's getting all the traffic and construction and event information along my route. So it's really good at telling me right now. Don't take that route or you're going to be 15 minutes late to your meeting. Right you get in your car you can it's pretty accurate. You know that's good. And the app itself is going to get that information and you know, I'm old enough I remember the days when you had you know I lived in Southern California you had a Thomas guide this like that. A 300 page map of this, you know, incredibly large Los Angeles metropolitan area, and you had no idea, you know, two miles into your drive, if there was construction or traffic or, you know, a police car and an accident, now, it's gathering all that information for you, you know, that's not really advanced machine learning. But that's an example of where the job gets done for the customer. That that doesn't require the customer to go find the information and analyze it and process it. Now, you get to more sophisticated jobs, or or I should say, you know, different jobs. And not not really sophistication judgments. But at the tribunal
Jared Ranere:sales is pretty sophisticated. Yeah, that's right.
Jay Haynes:So it but if you're thinking about, you know, optimizing cash flow in a company, or if you're thinking about coronary artery disease, or you know, knee surgery, the information that you need to get, and process, you know, pre op, post op, during surgery, you know, etc, all of these signals are going to be interpreted by these machine learning and AI to assist a surgeon or a patient, or a CFO, or salesperson, so that those decisions and those information that is the extraordinary power of having a information gathering and processing system that helps the customer get the job done better. And a great example of this in a technical field is copilot, which is chat GPT, in GitHub, to create code. And I think everybody's, you know, rightly or wrongly focused on Google and Bing, and the crazy mistakes they're making in the moody teenager, you know, perspective. But I think, and all the biases, and all those things are certainly important, and they need to be improved. They need to be fixed. And they are they can be dangerous, and all the caveats and the risk with that. But what I think is extraordinary is what's happening with copilot and GitHub just launched copilot for business. And I think this code creation, because it lacks all of the problems of the biases of chat GPT, meaning it might be biased between Python or Java, but that doesn't matter at all. It may matter to you know, humans who like Java or Python, but those kind of code debates or just religious debates, all it matters from a code perspective is does it work? Does it actually function to get the job done in the way that we want it to do? And that, that productivity increase in that ability of chat GPT to complement teams and assist in that kind of development, productivity is just going to be extraordinary, all of the equity value in software is going to be created by this combination of assisted AI machine learning code, plus, being able to get the job done for the customer faster and more accurately, that that's a prediction I'm willing to make is that over the next, you know, 10 years, you're gonna see this just extraordinary equity value creation, because these two things are coming together to get jobs done faster and more accurately.
Jared Ranere:Yeah, and in case you haven't seen it out there. Copilot enables you to as far as I understand it, J and correct me if I'm wrong finish lines of code, because you'll start writing it and then chat GPT will predict the rest of the code that you're about to write. And so it just it literally saves you keystrokes. Is that right? Or can you actually well, it can create
Jay Haynes:tire base. Yeah, it can create code based on prompts. So prompt engineering is becoming a job. I mean, there's there's a new job category of people who can create these prompts for co pilot in order to generate sophisticated amounts of code, and that is going to accelerate. So I think the other thing that's hard for humans to get their minds around because we didn't evolve to confront exponential growth. You know, the only time we really would confront that, in our evolutionary history is, is viruses. And you know, even even that Coronavirus, shows that just in the modern world, the explosion of viral growth is a lot because of where this late stages of industrialization where everybody's connected. So, we don't really have an inherent ability to understand what it means to confront exponential growth. Some companies do and they capture enormous equity value. And my favorite example of this is Gmail. When Gmail launched, they decided to give everybody a gigabyte of free storage and to put All Mail in the cloud. And you may remember, you know, I'm old enough where you were dealing with POP or IMAP, and then you had your big email file, and you had to download it to your local machine, it was a huge pain. And if you wanted to save your emails, which you may do for work, or personal, you know, to have this kind of archive of things you might want to search, it was really, really hard. And Google said, we're just putting all it seems so obvious today. But when they did it, people said it's gonna bankrupt, you know, Google is gonna be so expensive, because the price of storage, well, what they saw was essentially an exponentially dropping cost per megabyte of storage, and they just projected out well, okay, it's, it's going to ask them topically approach zero for us as a cost to store all this data. And what we will be able to do with that data is going to be extraordinary. And, and they were ahead of the curve. And of course, you know, I love I just went to a doctor who's still using an AOL address, which I thought was, you know, very impressive, but most people are using, you know, the free online email systems in that are all entirely stored in the cloud. And that's just that's a simple example. But you have to take advantage of these curves, because this stuff is getting better at an extraordinary rate.
Jared Ranere:Right. And I think we've seen that right. So you know, I, we've been playing with GPT, three, the API launched by open AI, which is the large language model that we're using, right, and that we started that about, I think, a year ago, maybe a few months ago, chat GPT is based on GPT 3.5, they're going to come out with with GPT. Four soon. And it's getting significantly better with each release, like radically better. And so I think this raises a couple of questions to capture this exponential value you, you have to think about the applications you can create, and also the platforms you can create. So the API is the platform, right? So even copilot. If you're using copilot, you're still enabling open AI to win in this race to create equity value or in large language models, because it uses open API's API's. So you're giving money to open API, open AI every time you do it. Chat. GPT is an application that open AI built on top of that, if you're Google, they seem to be taking an approach where they're going to and I don't entirely know yet. But they'll launch an API on their large language model, which is a different model than open AI is using. And they'll also create applications like Bard, and they'll put their large language model into their own application. So we'll try to compete on both fronts. Microsoft has said, we're just going to invest capital in open AI. And not really, as far as I can tell, play in the arms race to win the platform game. But we will play in the application game by putting open API's API in the back of Bing, so that you can get chat like really large language model responses in the search engine. So how do we unpack that? If you're if you're like, imagine your Amazon, what do you do? Do you compete on the platform level? Do you complete an application level? Do you compete on both? And if you're a startup, how do you think about that?
Jay Haynes:Yeah, I think that the great questions, I think you're right, that the application layer drives the equity value, because just having, you know, machine learning, or AI, we've talked about this in another podcast, it's not enough, it has to get the job done. And it has to be faster and more accurate. So, you know, the current state is it's very fast, maybe not so accurate. So there, there's some things to work on there. But I think you're right, the application layer is where you your customer facing and your customer centric, because your customer at the end of the day, I don't care how Apple and Google Maps get their traffic data, if it's AI or machine learning, or they put a human on every corner of every street in my city and just have the guy you know, phone it in. I don't care. In fact, Waze did that, right? Waze. Had user reported data, they were like, Hey, why don't you just you know, you get points in our little traffic game, if you report that there's a traffic slowdown. So the the underlying way that the data gets there and gets processed, the end user does not care that it doesn't matter. It has to get me to my destination on time and the example of Waze or Google Maps. Now, I will say there's a risk, especially for these bigger companies, you know, Google and Microsoft, and Amazon. You don't want to be so big and be using someone else's underlying technology. So over time, you can use someone's API certainly if you're smaller companies if you're a private equity backed company, If you're trying to grow from 25 to 250 million, or even if you're a$250 million company trying to grow to a billion there, you should not try to be duplicating open API's efforts, you should build the application layer. And that's a pretty good investment thesis. If you are Microsoft, now, there's a probability, which I would say is probably very high that Microsoft bought buys, you know, open AI at some point, because they want to differentiate on the technology layer, and you can, anybody who's got that big of a platform, likely is going to want to own the underlying technology, even Apple, the extreme case of Apple, they now build their own chips. I mean, it's, it's, it's remarkable to think about, you know, when I was in business school, you would talk about vertically integrated companies and vertically integrated companies were just, you know, terrible, because they couldn't optimize each level of the production and cores, I think was always used as the example of the extreme vertically integrated company, like they, I think they grew their own wheat. And then you know, the entire process of going from seed to beer in you're at it in front of you in a bar was like owned by Coors. It was a weird, odd company. Right. And, you know, and Apple obviously outsources a bunch of this to, you know, the manufacturing is done by, you know, other companies they own, you know, the piece of it, but the core technologies, they really own
Jared Ranere:it. Now, we just said quickly and interesting counter example to this is an evidence of why it can go wrong, if you're a large platform is when target first got into E commerce, they had their webs, the engine running their website was Amazon's e commerce engine, right? They use, they licensed Amazon's technology, you could read about this in The Everything Store the book about Amazon. And then at a certain point, Amazon just pulled the plug on target, and set them way back, I think they give them some warning, when it was going to happen. So Amazon could try to build something to stand it up before the contract ran out. But you know, look at the market capitalization of Amazon versus target. And you'll see how well that strategy went for target, which was much larger at the time that Amazon was powering their website.
Jay Haynes:Yeah, and that's a very interesting analysis, because Joss Whedon helps there, if you were analyzing that, basically, Amazon and target, were targeting the exact same customers with the exact same job in retailing. So that's a situation where you would not want to hand something as valuable as your development of your E commerce, which was clearly going to grow, right, if you just looked at speed and accuracy, people are gonna order stuff online, you know, I'm having to drive to the store and shop around the store is just, you know, for an enormous number of products, that's a huge waste of time. So you could tell that that was a mistake. And I think at the platform levels, whether you're, you know, Apple, Amazon, you know, Google whoever, you know, big companies, they should be building this technology on their own. Now, if you're a private equity company, and you're trying to create equity value for your shareholders, the decision should be to integrate this, the AI technologies, where it's going to help your customers get their job done faster and more accurately, and your, your solution is going to do that for them. But you can also take into consideration that you're sending data out to the API's of whatever API point machine learning platform you want to use. And you can switch, right, there are going to be multiple of them. And you can say, Okay, now, it's not an easy thing to do, of course, and building that kind of switch ability into your platform is non trivial, but you wouldn't be caught off by the knees, like Target was by Amazon. You can decide, hey, I which one of these platforms is going to be better for me and you can use it, you could switch from AWS to, you know, Google Cloud or as your if you want to write it wouldn't you know, it's again, not trivial, but you know, you could do it.
Jared Ranere:Yeah. And I think Google Cloud and Azure and AWS are good analogies for the platform nature of open eyes business, right. So they have this consumer application chat, GBT. But then if you look at it from their API perspective, their customers are developers who are trying to develop software, you know, AI driven software that satisfies needs for their customers. Just like, you know, AWS and GCP, and Azure providing hosting services to developer ops are called CTOs as their customer. And so you can still those companies can be using Jobs-to-be-Done to better understand their customers and you can start to predict where the edges are going to be created the advantages and differentiation by the companies developing large language models and selling API access to it. So there's there's competition to be had There, I have for years, I've heard people liken AI to electricity, where you know, it's just gonna be powering everything in the background. And maybe someday it gets regulated, you have to abide by certain guidelines. And you can only make a certain amount of profits, just like utility companies can, if you are an AI platform, we're so far from that there's a ton of equity value to be created by looking at developers as your customers and playing that game to figure out how to deliver more value to developers to get them to use your API.
Jay Haynes:Yeah, I think that's right. And if the question is, how would you beat GPT? You know, it is to create applications that help people get the job done that use chat GPT. Now, if you're a Google, and they're your direct competition for these AI platforms, you have to be faster and more accurately, you know, that's, that's the key. And they're both they both have a lot of work to do on this, obviously. But if you're looking to create equity value, in, you know, private companies in, you know, your new venture, whatever it is, it's still to be customer focused, you have to figure out what problem you're solving with machine learning or AI. First and foremost, because that is where all equity value is created over time. At the end of the day, you're selling to humans, somewhere, there's a person, whether in a professional or, or a personal consumer or medical role that has to get the job done, and they're willing to pay for some solution that does that better. And that's what, that's what's so exciting about this, it's going to be you know, a wild ride over the next few years. But there will be enormous equity value creation, my so I would estimate that it's going to be it's going to dwarf the equity value created by the Internet. Because the internet connected everybody and enabled applications to be connected. And it did solve problems and got jobs done faster and more accurately, right? You go to Google instead of a library and Encyclopedia Britannica, that's, you know, with enormous speed and accuracy increase, but but it really is just looking at hundreds and hundreds of customer jobs over the past, you know, 1015 years. You can see into those. And this is where the real equity value is going to turn on. And, you know, part of it is because it's connected to the internet, obviously. But it could be just an extraordinary equity value creation time.
Jared Ranere:Yeah, I agree. It's yeah, it's very exciting. So whether you're building an application and understanding the needs of your customers or businesses that are your customers, or building a platform, understanding the needs of developers, I think Jaspreet is going to be a helpful way to understand how to capture that value.
Jay Haynes:Yeah, I think that's right. Well, that's a good place to end. Thanks for listening. Remember to subscribe and like to this podcast. If you want to learn more about jobs done innovation methods visit us at Thrive t h r v.com.